The Role of AI in Predicting and Preventing Natural Disasters
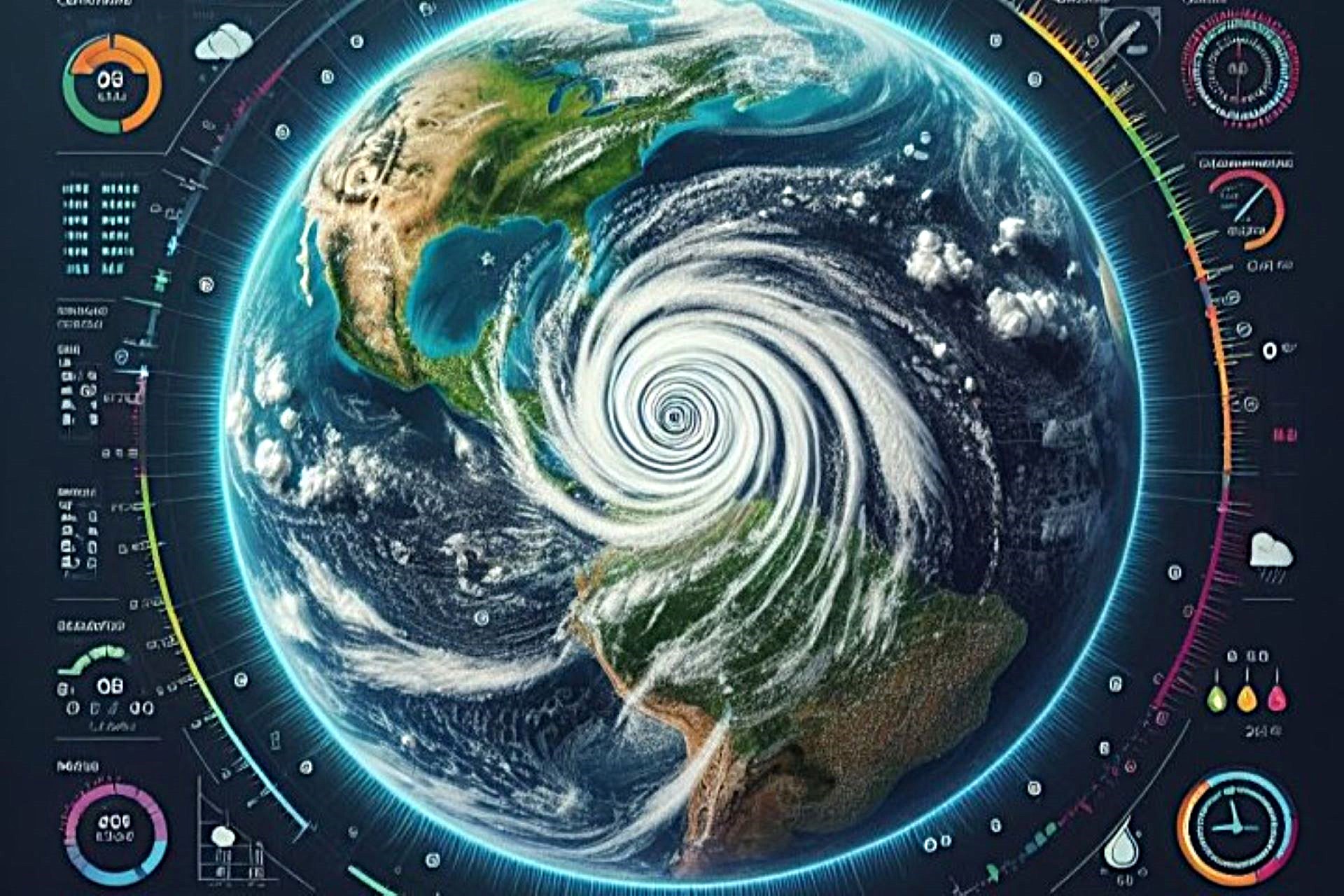
The threat posed by natural disasters is ever-present, with events such as earthquakes, hurricanes, floods, and wildfires causing significant damage to lives and property every year. Traditional methods of predicting these disasters have often been limited in their accuracy and timeliness. However, with the advent of Artificial Intelligence (AI), there is a growing potential to revolutionize how we predict and prevent these catastrophic events. AI’s ability to process vast amounts of data and identify patterns that may not be immediately apparent to human analysts makes it a powerful tool in disaster management.
Understanding Natural Disasters
Types of Natural Disasters
Natural disasters can be broadly categorized into several types, each with unique characteristics and impacts:
- Geological Disasters: Earthquakes, volcanic eruptions, and tsunamis.
- Meteorological Disasters: Hurricanes, typhoons, tornadoes, and thunderstorms.
- Hydrological Disasters: Floods, landslides, and avalanches.
- Biological Disasters: Epidemics, pandemics, and infestations.
- Climatological Disasters: Droughts, wildfires, and extreme temperatures.
Each type of disaster requires specific predictive models and preventive strategies, making the role of AI in this field both complex and diverse.
The Importance of Prediction and Prevention
Predicting natural disasters with accuracy is vital for taking preemptive measures to protect lives and infrastructure. Prevention, on the other hand, involves mitigating the impact of these disasters through strategic planning and preparedness. Together, prediction and prevention are the cornerstones of effective disaster management. AI enhances our ability to predict disasters by analyzing large datasets and providing more accurate forecasts, which in turn, informs better preventive measures.
The Evolution of AI in Disaster Prediction
Early Attempts and Limitations
Before AI, disaster prediction relied heavily on historical data, statistical models, and basic computational tools. While these methods provided some insights, they often fell short in predicting the exact timing, location, and severity of disasters. For example, earthquake prediction has traditionally been based on seismological data, but the ability to predict when and where an earthquake will strike has been limited.
The Emergence of AI and Machine Learning
The introduction of AI and machine learning has significantly improved the accuracy of disaster predictions. Machine learning algorithms can process vast amounts of data from various sources, including satellite imagery, sensor networks, and historical records. These algorithms can identify patterns and correlations that may not be apparent through traditional methods. As a result, AI has opened new avenues for more accurate and timely predictions.
How AI Predicts Natural Disasters
Data Collection and Analysis
AI relies on large datasets to make accurate predictions. Data is collected from various sources, including:
- Satellites: Provide real-time images and climate data.
- Sensor Networks: Gather data on environmental conditions such as temperature, humidity, and seismic activity.
- Social Media: Crowdsourced information on emerging disaster situations.
- Historical Records: Past disaster data helps in pattern recognition and model training.
AI systems analyze this data to identify patterns and predict potential disaster events.
Machine Learning Algorithms in Prediction
Machine learning (ML) algorithms are at the core of AI-based disaster prediction. These algorithms can be categorized into several types:
- Supervised Learning: Uses labeled datasets to train models for predicting specific disaster types.
- Unsupervised Learning: Identifies unknown patterns in data, useful in discovering new predictive indicators.
- Reinforcement Learning: Continuously improves prediction models based on new data inputs and outcomes.
These algorithms can predict various aspects of natural disasters, such as their location, timing, and intensity, with greater accuracy than traditional methods.
Real-Time Monitoring and Early Warning Systems
AI-driven real-time monitoring systems are vital in providing early warnings for natural disasters. These systems use AI to continuously analyze data from sensors and other sources, providing alerts when certain thresholds are exceeded. For example, AI-powered seismic sensors can detect early tremors, triggering an earthquake alert system. Similarly, AI can analyze weather patterns to predict hurricanes and provide timely warnings to affected regions.
Case Studies of AI in Natural Disaster Prediction
Earthquakes
Earthquakes are notoriously difficult to predict, but AI has made significant strides in this area. Machine learning algorithms can analyze seismic data to identify patterns that precede earthquakes. For instance, AI has been used to detect minor tremors that often occur before major quakes, allowing for early warnings that can save lives.
Hurricanes and Typhoons
AI is playing a crucial role in predicting the path and intensity of hurricanes and typhoons. By analyzing satellite images, atmospheric data, and historical storm patterns, AI models can provide accurate forecasts days in advance. This allows for better preparation and evacuation planning, reducing the potential loss of life and property.
Floods
Flood prediction has traditionally relied on meteorological data and river levels. AI enhances this process by incorporating real-time data from sensors and satellite images. Machine learning models can predict the likelihood of flooding in specific areas, helping authorities issue timely warnings and implement flood prevention measures.
Wildfires
AI is increasingly being used to predict and prevent wildfires. Machine learning algorithms analyze data on vegetation, temperature, humidity, and wind patterns to predict the risk of wildfires. AI can also help in monitoring active fires and predicting their spread, aiding firefighting efforts and resource allocation.
AI in Disaster Prevention
Infrastructure Planning and Development
AI plays a critical role in disaster prevention through its application in infrastructure planning and development. By analyzing data on various factors, AI can help in designing infrastructure that is more resilient to natural disasters. Some key applications include:
- Soil Composition Analysis: AI can evaluate soil stability to determine the safest locations for construction.
- Weather Pattern Monitoring: Predicting weather conditions helps in designing infrastructure that can withstand extreme climates.
- Historical Disaster Data: AI uses past disaster events to guide the design of disaster-resistant infrastructure.
- Location Selection: AI determines the best locations for constructing flood-resistant buildings.
- Earthquake-Resistant Design: AI aids in the creation of structures that can endure seismic activity.
Mitigation Strategies
Mitigation involves reducing the impact of disasters when they occur. AI contributes to mitigation strategies by offering predictive insights that inform policy and planning. Key AI-driven mitigation strategies include:
- Flood Risk Identification: AI identifies areas at high risk of flooding.
- Levee Construction: AI supports the design and placement of levees to prevent flood damage.
- Land-Use Policies: AI informs the development of land-use policies to minimize disaster risks.
- Disaster Scenario Simulation: AI models potential disaster scenarios to test and improve mitigation strategies.
AI Contributions to Mitigation Strategies
Mitigation Strategy | AI Contribution | Benefit |
Flood Risk Identification | Analyze data to pinpoint flood-prone areas | Targeted flood prevention measures |
Levee Construction | Design and optimize levee placement | Enhanced flood defense |
Land-Use Policy Development | Inform land-use regulations to reduce risk | Safer land development |
Disaster Scenario Simulation | Model potential disaster outcomes | Improved preparedness and response planning |
Enhancing Community Resilience
AI also plays a role in enhancing community resilience to natural disasters by ensuring that communities are well-prepared and can respond effectively. Important contributions include:
- Evacuation Route Planning: AI provides the most efficient evacuation routes during emergencies.
- Public Safety Information: AI disseminates timely information on safety procedures and emergency resources.
- Emergency Resource Allocation: AI helps prioritize resources for disaster response.
- Damage Assessment: After a disaster, AI assesses damage to prioritize recovery efforts.
- Post-Disaster Recovery: AI aids in organizing and executing recovery operations more efficiently.
The Challenges of Using AI in Disaster Management
Data Limitations and Quality
One of the significant challenges in using AI for disaster management is the quality and availability of data. Accurate predictions depend on high-quality, up-to-date data from various sources. In some regions, particularly in developing countries, the lack of reliable data can limit the effectiveness of AI-based disaster prediction models.
Ethical Considerations
AI in disaster management raises several ethical considerations. For instance, decisions based on AI predictions can have significant impacts on communities, such as evacuation orders or resource allocation. Ensuring that AI systems are transparent, fair, and free from biases is crucial in maintaining public trust and ensuring ethical use.
Technological and Infrastructural Challenges
The implementation of AI in disaster management requires significant technological and infrastructural investments. This includes deploying sensor networks, maintaining data storage systems, and ensuring that AI models are regularly updated and validated. In resource-limited settings, these challenges can be a barrier to the effective use of AI in disaster management.
The Future of AI in Predicting and Preventing Natural Disasters
Advances in AI Technologies
The future of AI in disaster management is promising, with continuous advances in AI technologies. Developments in deep learning, natural language processing, and neural networks are expected to enhance the accuracy and reliability of disaster predictions. These advances will likely lead to more sophisticated models that can predict a wider range of disaster scenarios with greater precision.
The Integration of AI with Other Technologies
The integration of AI with other emerging technologies, such as the Internet of Things (IoT), remote sensing, and blockchain, is likely to revolutionize disaster management. For instance, IoT devices can provide real-time data to AI systems, enhancing predictive capabilities. Blockchain can ensure the transparency and security of data used in AI models, addressing some of the ethical concerns associated with AI.
Policy and Global Collaboration
Global collaboration and policy development will play a critical role in the future of AI in disaster management. International cooperation can facilitate data sharing, enhance technological capabilities, and establish global standards for the ethical use of AI in disaster management. Policies that support research and innovation in AI, while ensuring its ethical application, will be crucial in maximizing the benefits of AI in this field.